Third edition of Artificial Intelligence: foundations of computational agents, Cambridge University Press, 2023 is now available (including the full text).
6.5.4 Dynamic Belief Networks
You do not have to represent the state at a particular time as a single variable. It is often more natural to represent the state in terms of features.
A dynamic belief network (DBN) is a belief network with regular repeated structure. It is like a (hidden) Markov model, but the states and the observations are represented in terms of features. Assume that time is discrete. If F is a feature, we write Ft as the random variable that represented the value of variable F at time t. A dynamic belief network makes the following assumptions:
- The set of features is the same at each time.
- For any time t>0, the parents of variable Ft are variables at time t or time t-1, such that the graph for any time is acyclic. The structure does not depend on the value of t (except t=0 is a special case).
- The conditional probability distribution of how each variable depends on its parents is the same for every time t>0.
Thus, a dynamic belief network specifies a belief network for time t=0, and for each variable Ft specifies P(Ft|parents(Ft)), where the parents of Ft are in the same or previous time step. This is specified for t as a free parameter; the conditional probabilities can be used for any time t>0. As in a belief network, directed cycles are not allowed.
The model for a dynamic belief network can be represented as a two-step belief network that represents the variables at the first two times (times 0 and 1). That is, for each feature F there are two variables, F0 and F1; parents(F0) only include variables for time 0, and parents(F1) can be variables at time 0 or 1, as long as the resulting graph is acyclic. Associated with the network are the probabilities P(F0|parents(F0)) and P(F1|parents(F1)). Because of the repeated structure, P(Fi|parents(Fi)), for i>1, has exactly the same structure and the same conditional probability values as P(F1|parents(F1)).
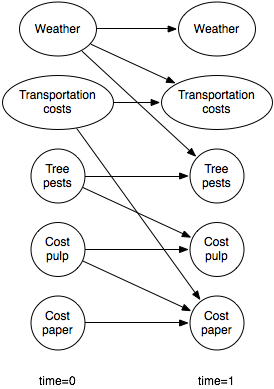
Note that, in this figure, the variables are initially independent.
This two-stage dynamic belief network can be expanded into a regular dynamic belief network by replicating the nodes for each time step, and the parents for future steps are a copy of the parents for the time 1 variables.
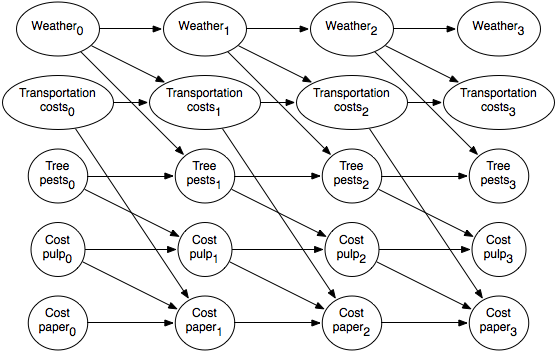
An expanded belief network is shown in Figure 6.20. The subscripts represent the time that the variable is referring to.